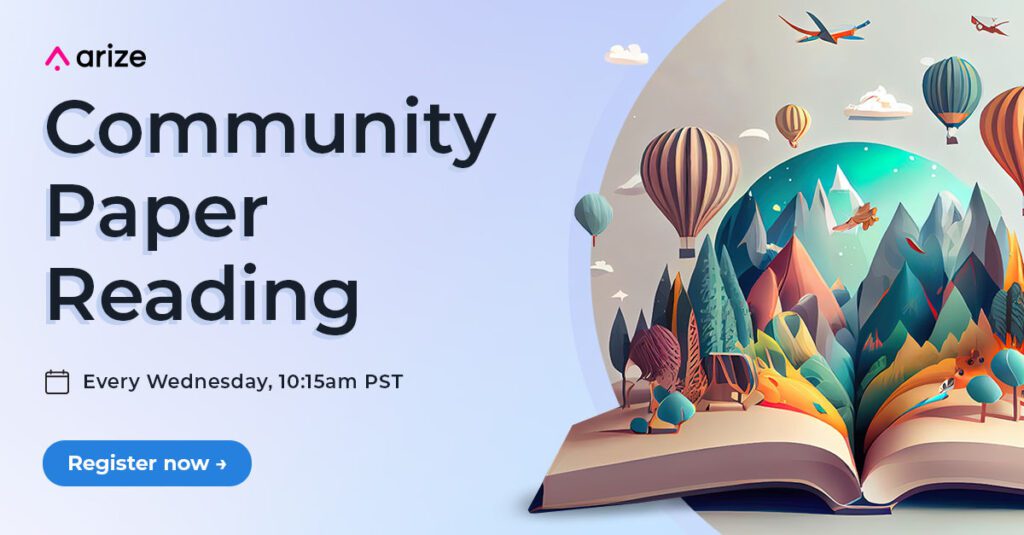
Live | Every Wednesday
10:15am PT | 45 minutes
Join us every Wednesday for an engaging discussion session where we delve into the latest technical papers, covering a range of topics including large language models (LLM), generative models, ChatGPT, and more. This recurring event offers an opportunity to collectively analyze and exchange insights on cutting-edge research in these areas and their broader implications.
Introducing GLoRA: a universal, parameter-efficient fine-tuning approach for diverse tasks. GLoRA enhances LoRA with a generalized prompt module, optimizing pre-trained model weights and activations. Its scalable, layer-wise structure search enables efficient parameter adaptation. GLoRA excels in transfer learning, few-shot learning, and domain generalization, outperforming previous methods on various datasets. With fewer parameters and no extra inference cost, GLoRA is a practical solution for resource-limited applications. Join us to explore GLoRA’s capabilities in this interactive community paper reading!
Link to Paper: https://arxiv.org/abs/2306.07967
Recent research focuses on improving smaller models through imitation learning using outputs from large foundation models (LFMs). Challenges include limited imitation signals, homogeneous training data, and a lack of rigorous evaluation, leading to overestimation of small model capabilities. To address this, we introduce Orca, a 13-billion parameter model that learns to imitate LFMs’ reasoning process. Orca leverages rich signals from GPT-4, surpassing state-of-the-art models by over 100% in complex zero-shot reasoning benchmarks. It also shows competitive performance in professional and academic exams without CoT. Learning from step-by-step explanations, generated by humans or advanced AI models, enhances model capabilities and skills.
Link to Paper: https://arxiv.org/abs/2306.02707
Explore HyDE, a thrilling zero-shot learning technique that combines GPT-3’s language understanding with contrastive text encoders. HyDE revolutionizes information retrieval and grounding in real-world data by generating hypothetical documents from queries and retrieving similar real-world documents. It outperforms traditional unsupervised retrievers, rivaling fine-tuned retrievers across diverse tasks and languages.
This leap in zero-shot learning efficiently retrieves relevant real-world information without task-specific fine-tuning, broadening AI model applicability and effectiveness. Join us for a paper reading on how HyDE works!
Link to Paper: https://arxiv.org/abs/2212.10496
Recording: https://youtu.be/PvT8ntmm1Xs
VOYAGER, the first LLM-powered embodied lifelong learning agent in Minecraft, autonomously explores the world, acquires skills, and makes discoveries without human intervention. It outperforms previous approaches, achieving exceptional proficiency in playing Minecraft and successfully applies its learned skills to solve novel tasks in different Minecraft worlds, surpassing techniques that struggle with generalization.
Link to Paper: https://arxiv.org/pdf/2305.16291.pdf
Link to Recording: https://www.youtube.com/watch?v=BU3w_AbCEbA
This week we’re diving into the world of Retrieval-Augmented Generation (RAG)!
We know GPT-like LLMs are great at soaking up knowledge during pre-training and fine-tuning them can lead to some pretty great, specific results. But when it comes to tasks that really demand heavy knowledge lifting, they still fall short. Plus, it’s not exactly easy to figure out where their answers come from or how to update their knowledge.
Enter RAG models, a hybrid beast that combines the best of both worlds: the learning power of pre-trained models (the parametric part), and an explicit, non-parametric memory — imagine a searchable index of all of Wikipedia.
Link to paper: https://arxiv.org/abs/2005.11401
This paper introduces a novel approach, DragGAN, for achieving precise control over the pose, shape, expression, and layout of objects generated by GANs. It allows users to “drag” any points of an image to specific target points — in other words, it enables the deformation of images with better control over where pixels end up to produce ultra-realistic outputs. Paper: https://arxiv.org/abs/2305.10973
View Recording: https://youtu.be/DxzsgV8rTOw